How to finetune the t5-small model to generate colour hex - Part 1
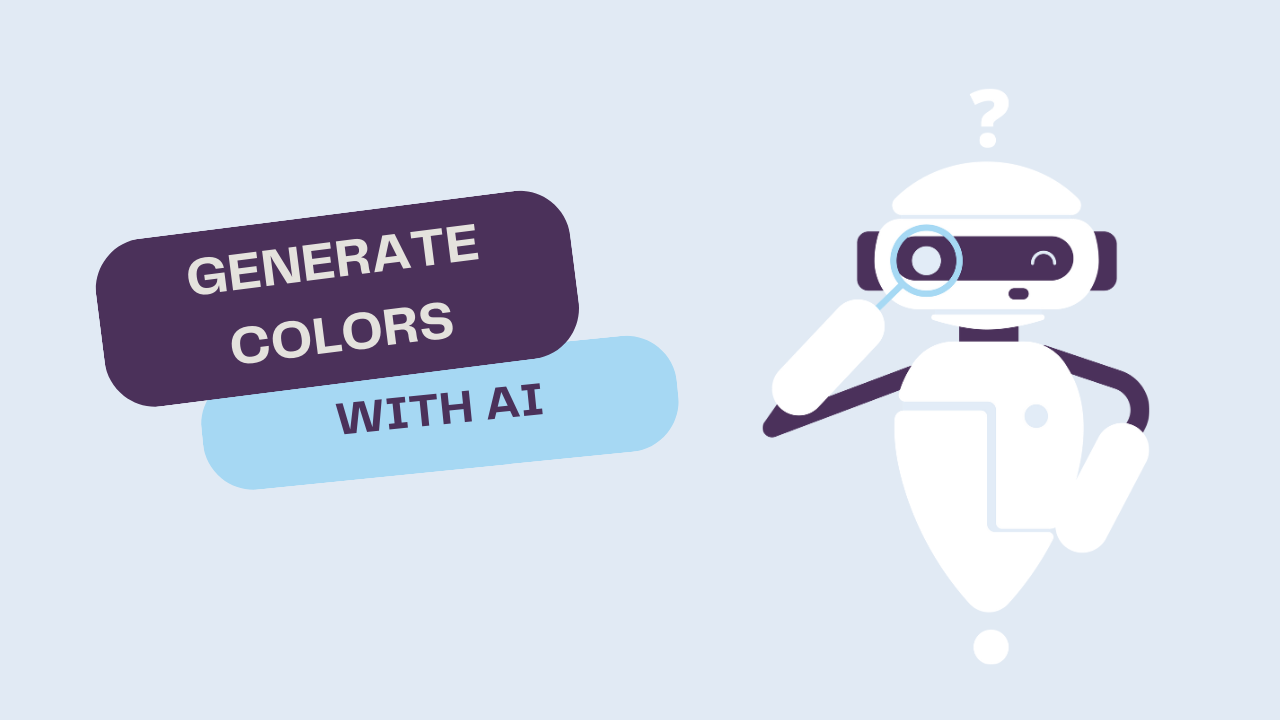
This was my first LLM to be finetuned, so I wanted to take on a relatively simple task.
Before starting, we need to draft a plan to show how this model will be finetuned; the result here was to generate a 4-colour palette using user input, and the output will be a 4-colour hex.
This post is one of a series of posts on how to fine-tune a model.
The Plan
- Find a model that already does that
- Curate/Find a dataset
- Prepare the data
- Train the model
- Evaluate and reiterate until we get the best results (Size is essential, not always)
The Search
As the plan was clear and with the first step, I started my journey of hugging faces to find a model that already does that. I give it some text, and it returns with a hex colour or any colour representation. After a couple of hours, it seemed that it wasn’t an easy ask; I wasn’t able to find a model that specialized in that sort of task (Is it colour or colour)
That means we need to find a generic model that can be used to fine-tune it to the task. The criteria for picking a model are that it should be small and contain enough data to tokenize the input and label; t5-small will be the picked model. The next step will be to collect/find the data used in the training process.
The Data
The second step was to find data on whether a model still needed to exist to start the finetuning process. Again, it was not an easy task; as I was looking for text to 4 hex colours, I couldn’t find such data on hugging faces or in my search in general. So I rolled my sleeves, headed to a chatbot, and asked, Suggest a dataset to finetune an LLM to provide 4 colours when given a palette name,
here is the output.
| Palette Name | Color 1 | Color 2 | Color 3 | Color 4 |
|-----------------|------------|------------|------------|------------|
| Spring Meadow | #8FD400 | #62C100 | #3AA300 | #207C00 |
| Ocean Blues | #007BFF | #0056B3 | #003D66 | #001F33 |
| Sunset Shades | #FF4500 | #FF7E00 | #FFAC00 | #FFD700 |
| Autumn Hues | #FFA500 | #D2691E | #8B4513 | #8B0000 |
| Pastel Dream | #FFD700 | #FFA07A | #FFB6C1 | #87CEEB |
It looks promising, but it could be better to ask ChatGPT to generate a couple hundred. I just ended up having lots of duplicates. There has to be another solution.
The Scrapping
I love scrapping; it’s one of the tools every developer should have; through scrapping, you get exposed to different kinds of tech through the target websites. I explored a couple of colour palette websites and started to look for ones that provide both a colour palette and theme name or a description of those colours.
I finally found one of the websites that does that and compiled around ~800 rows.
import requests
from bs4 import BeautifulSoup
import json
color_palettes = []
for i in range(1, 800):
URL = "https://thewebsite" + str(i) + "/"
page = requests.get(URL)
soup = BeautifulSoup(page.content, "html.parser")
results = soup.select("#colors")
## Loop through all the colors and store them in an array
colors = []
for result in results:
colors.append(result['data-clipboard-text'])
## if no colors, skip
if len(colors) == 0:
continue
description = soup.select_one(".text_content p").text
tags = soup.select_one(".tags").text
## add to object
color_palettes.append({
"colors": colors,
"description": description,
"tags": tags
})
##Saving every iteration to avoid issues and losing all data
with open('color_palettes.json', 'w') as outfile:
json.dump(color_palettes, outfile)
Data Prep
Now that we have some data, data preparation is one of the critical steps in fine-tuning and training in general. I will be using it. datasets
from datasets import load_dataset,concatenate_datasets
dataset = load_dataset("json",data_files = "color_palettes.json")
This will load the data into the dataset object. Now, let’s split the data into train and test.
dataset = dataset.train_test_split(test_size=0.1)
This will split the dataset into 2, 90% training and 10% testing
# Define our preprocessing function
def preprocess_function(data):
# The "inputs" are the tokenized text:
inputs = [ desc.strip() for desc in data["description"]]
model_inputs = tokenizer(inputs, max_length=350, truncation=True, padding=True)
colors_input = [' '.join(colors) for colors in data["colors"]]
# The "labels" are the tokenized outputs:
labels = tokenizer(text_target=colors_input, max_length=100, truncation=True, padding=True)
model_inputs["labels"] = labels["input_ids"]
return model_inputs
# Map the preprocessing function across our entire dataset
tokenized_dataset = dataset.map(preprocess_function, batched=True)
This preprocessing will trim any spaces and tokenize both inputs and labels (outputs). Here, the data is ready for training.
The Training
Once we have all the data ready, we can start configuring our trainer and begin the training process.
First, we need to configure our evaluation function.
nltk.download("punkt", quiet=True)
metric = evaluate.load("rouge")
def compute_metrics(eval_preds):
preds, labels = eval_preds
# decode preds and labels
labels = np.where(labels != -100, labels, tokenizer.pad_token_id)
decoded_preds = tokenizer.batch_decode(preds, skip_special_tokens=True)
decoded_labels = tokenizer.batch_decode(labels, skip_special_tokens=True)
# rougeLSum expects newline after each sentence
decoded_preds = ["\n".join(nltk.sent_tokenize(pred.strip())) for pred in decoded_preds]
decoded_labels = ["\n".join(nltk.sent_tokenize(label.strip())) for label in decoded_labels]
result = metric.compute(predictions=decoded_preds, references=decoded_labels, use_stemmer=True)
return result
Here, we use ROUGE (metric) - Wikipedia as the evaluation metric.
Next, we will configure our training as follows.
training_args = Seq2SeqTrainingArguments(
output_dir="./results",
evaluation_strategy="epoch",
save_strategy="epoch",
learning_rate=3e-4,
per_device_train_batch_size=32,
per_device_eval_batch_size=32,
weight_decay=0.01,
num_train_epochs=10,
predict_with_generate=True,
push_to_hub=False,
load_best_model_at_end=True,
metric_for_best_model="rouge1",
)
In this config, we are storing the output in the results
folder, saving every epoch
, and our batch size will be 32
, our training epochs will be 10
, and we are saving the best model at the end using the rouge1
metric.
We will then load the base model, which we will fine-tune. This snippet will load the base model and tokenizer.
modelId = "t5-small"
tokenizer = AutoTokenizer.from_pretrained(modelId)
model = T5ForConditionalGeneration.from_pretrained(modelId)
data_collator = DataCollatorForSeq2Seq(tokenizer=tokenizer, model=model)
After the config, we will start the training process.
trainer = Seq2SeqTrainer (
model=model,
args=training_args,
train_dataset=tokenized_dataset["train"],
eval_dataset=tokenized_dataset["test"],
tokenizer=tokenizer,
data_collator=data_collator,
compute_metrics=compute_metrics
)
# Train the model
trainer.train()
Finally, saving the trained model, we are using time as a variable in the model output folder to avoid overriding a good model, as we will train multiple models and use the best one.
saved_dir = f'./model/trained-model/color-trained-{str(int(time.time()))}'
tokenizer.save_pretrained(saved_dir)
model.save_pretrained(saved_dir)
Testing
Testing this model has shown that the model was able to generate four hex colours. It is a good start, but it showed a lot of confusion and bais toward specific colours and constantly repeating colours in the output.
newModel = "./model/trained-model/color-trained-1703221585"
from transformers import AutoTokenizer, AutoModelForCausalLM, AutoModelForSeq2SeqLM
tokenizer = AutoTokenizer.from_pretrained(newModel)
model = AutoModelForSeq2SeqLM.from_pretrained(newModel)
input_ids = tokenizer("purple" , return_tensors="pt").input_ids
instruct_model_outputs = model.generate(input_ids=input_ids, generation_config=GenerationConfig(max_new_tokens=200, num_beams=1, do_sample=True))
instruct_model_text_output = tokenizer.decode(instruct_model_outputs[0], skip_special_tokens=True)
print(instruct_model_text_output) #ffffff #ffffff #ffffff #e2e2ee
In the next part of this series, we will review how to refine the process and improve the results.